AI-powered translation systems have revolutionized global communication. However, assessing the quality of AI translation remains critical to ensure these systems meet user expectations. Beyond traditional evaluation methods, several tools and utilities developed by vendors now assist in providing precise, scalable, and context-aware quality assessments. This article explores the current methods and tools shaping the landscape.
1. Human Evaluation
Human evaluation continues to be the gold standard in translation quality assessment. Professional linguists or domain experts analyze translations for fluency, accuracy, and cultural appropriateness. Popular approaches include:
- Direct Assessment (DA): Translators rate translations on a quality scale based on semantic equivalence and grammaticality.
- Post-Editing Effort (PEE): Measures the time and edits required to refine machine output into a publishable standard.
Many tools, like Memsource or Smartcat, integrate post-editing tracking to quantify efforts, providing valuable data for quality management.
2. Automated Metrics
Automated metrics are indispensable for scalability and rapid benchmarking, even if they cannot fully capture linguistic nuances. Key metrics include:
BLEU (Bilingual Evaluation Understudy): Measures n-gram overlaps between machine output and human references. It’s efficient but struggles with synonyms and flexible word order.
TER (Translation Edit Rate): Calculates the number of edits needed to transform machine-generated translations into reference-quality text.
METEOR (Metric for Evaluation of Translation with Explicit ORdering): Adds synonym matching, stemming, and word order considerations, improving semantic sensitivity over BLEU.
COMET and BERTScore: Advanced metrics built on neural networks, such as OpenAI’s GPT-based utilities and Google’s BERT models, offer superior evaluations of semantic similarity and context.
3. Vendor-Developed Tools and Plugins
Leading translation technology providers have developed specialized utilities to complement traditional evaluation techniques:
- Trados Studio QA Checker: Offers integrated quality assurance for translations, focusing on terminology consistency, formatting, and error detection.
- LQA Tools by XTM International: Enable detailed linguistic quality assessments (LQA) by tracking specific error types and generating actionable insights.
- TAUS MT Quality Estimation: This plugin assesses machine translation quality without requiring human reference translations. By analyzing factors like fluency and adequacy through AI algorithms, it provides real-time predictions on the usability of MT outputs. This is particularly valuable for large-scale workflows and pre-editing strategies.
- TAUS DQF Tools: Developed by the Translation Automation User Society, these tools provide industry benchmarks and real-time productivity metrics, linking error typology to automated scoring.
4. Error Typology and Quality Frameworks
Error typology is another important approach. The Multidimensional Quality Metrics (MQM) framework, supported by utilities like Memsource’s Quality Assurance module, enables precise classification of translation errors into categories like omissions, mistranslations, and stylistic issues.
5. End-User Feedback and Real-World Testing
Vendors increasingly prioritize tools that gather feedback from real-world use cases. Platforms like Unbabel provide a hybrid approach, combining AI translation with human-in-the-loop post-editing and end-user validation. Similarly, Lionbridge’s AI Translator Evaluator captures contextual insights from industry applications, ensuring tailored quality improvement.
Conclusion
Evaluating AI translation quality requires a multifaceted approach. While traditional methods like human evaluation and automated metrics remain essential, vendor-developed tools and plugins, such as TAUS MT Quality Estimation, significantly enhance scalability, precision, and actionable insights. By leveraging these advanced resources, organizations can ensure their AI translation systems deliver excellence in increasingly complex and diverse linguistic landscapes.
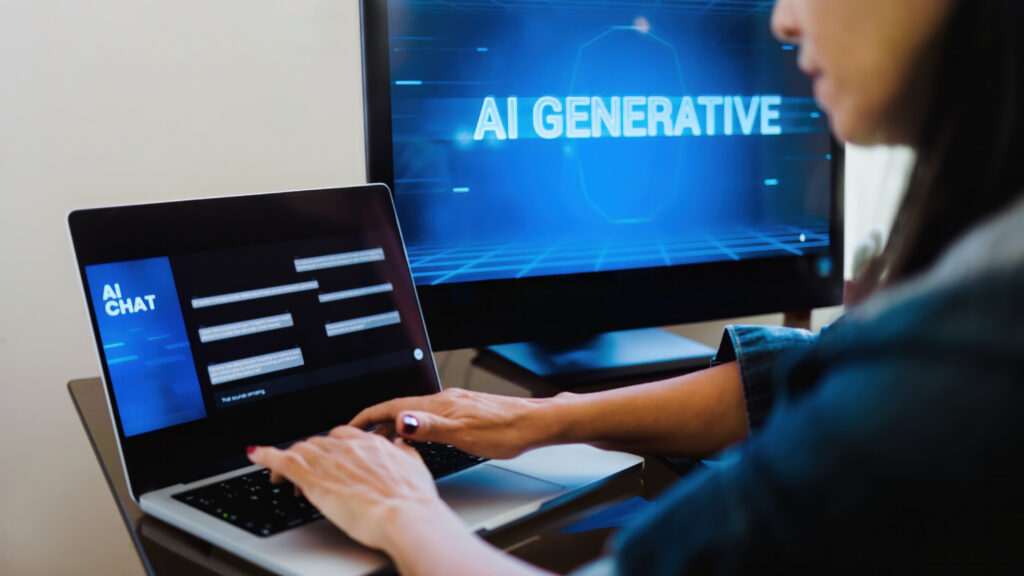